Ontology Basics
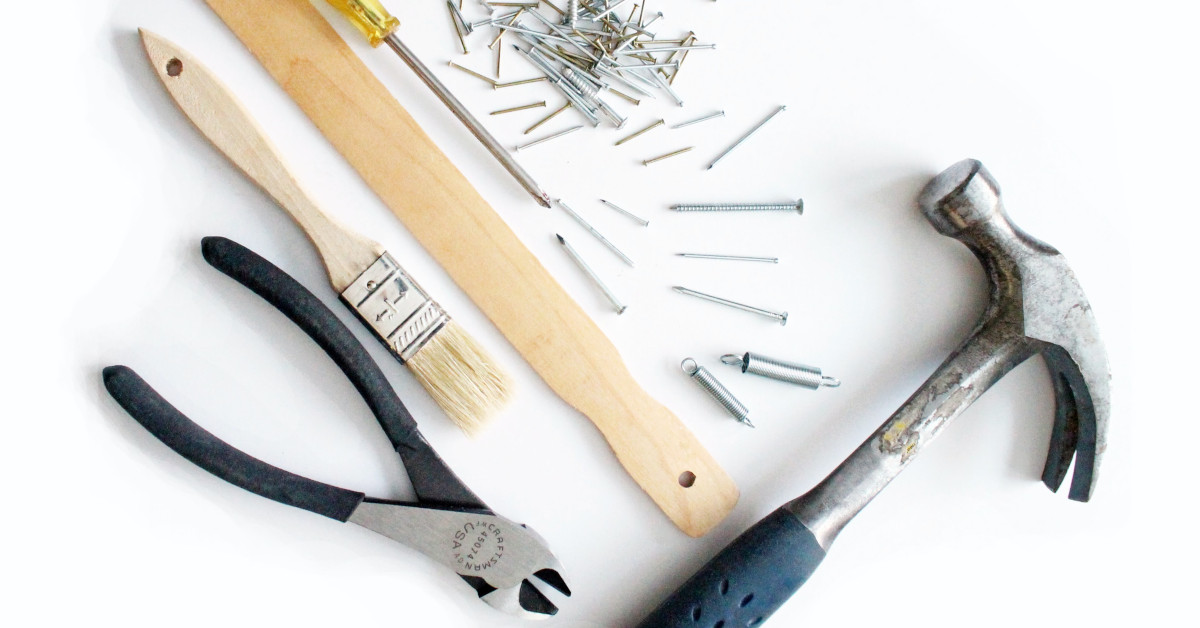
What are ontologies and why should I care? If you are a scientist and want your research to have long term impact, you will want to learn about ontologies and use them in your documentation.
We’ve talked previously about the importance of metadata in research data stewardship (Getting Started with FAIR). Connections between data sets from different assays can be strengthened by metadata – information on the compounds tested (e.g., which ones are associated with a particular adverse drug reaction), or the assays themselves (e.g., cell type used, or protein measured). Metadata annotation improves communication of research results and tools that support machine readability can help promote effective re-use of data.
Ontologies are important tools for metadata annotation. We can all better understand research results when we use the same words to mean the same thing. This is what ontologies are for – they are collections of standardized terms developed by experts in different domains.
A couple of things to keep in mind. There are loads of ontologies out there for the life sciences. With so many ontology efforts, it can be confusing. So below, we’ve gathered a few resources to help you get your terminology right.
Note that each ontology assigns their own unique identifiers for terms. Having these unique identifiers streamlines computational work and ensures accurate interpretation. Fortunately, there are sources that have collected terms from different ontologies so that they are easily cross-referenced.
EMBL-EBI Ontology Lookup Service is an excellent place to find terms from a variety of popular life science-based ontologies. Often terms are listed in several ontologies. For example, looking up “endothelial cell” gives you terms in the NCI Thesaurus (NCIT:C12865), Cell Ontology (CL:0000115) and the BRENDA Tissue Ontology (BTO:0001176) collections.
The NCBO BioPortal is another source that has collected and cross-referenced life science ontologies.
If you’re interested in the process of building an ontology, check out the OBO Foundry, a community that has developed best practices for building ontologies for the biological sciences. You might like to investigate ontology formats such as OWL (Web Ontology Language) or check out Protégé, an open source ontology editor.
For specific ontologies that are relevant to phenotypic assays and data analysis, there is no one size fits all. BioAssay Ontology has many of the relevant terms related to assay methods. However, it’s missing research reagents and does not contain cell types (cell lines, yes, but cell types, like “endothelial cell”, no).
The Gene Ontology (GO) is focused on genes, gene products and their functions. This ontology is used extensively in gene expression studies, for example to perform enrichment analysis on gene sets (for tools visit the GO Consortium).
The NCI Thesaurus includes reference terminology for biomedical coding and is particularly useful for clinical data and disease descriptions. Another good source for human disease terms is the Human Disease Ontology. This ontology was developed through collaborative efforts of biomedical researchers, coordinated by the University of Maryland School of Medicine, Institute for Genome Sciences.
Chemical Entities of Biological Interest (ChEBI) specializes in terms for molecular entities focused on ‘small’ chemical compounds. These include terms for drugs, metabolites, proteins and other biomolecules.
The Resource Identification Portal (RRID) is another useful site for specific research materials. This portal collates resource identifier collections. For example, a search for E-selectin and antibodies takes you to the Antibody Registrywhere you can find AB_2254464, the research identifier for the mouse anti-E-selectin IgG1 antibody, clone 1.2B6.
As you document your experiments, the more you can describe your processes using standardized terms, the easier it will be to connect your results to those from other labs. Using standardized terminology and persistent unique identifiers help meet the goal of FAIR data.
Photo by Julie Molliver on Unsplash.