Engineering in Biology
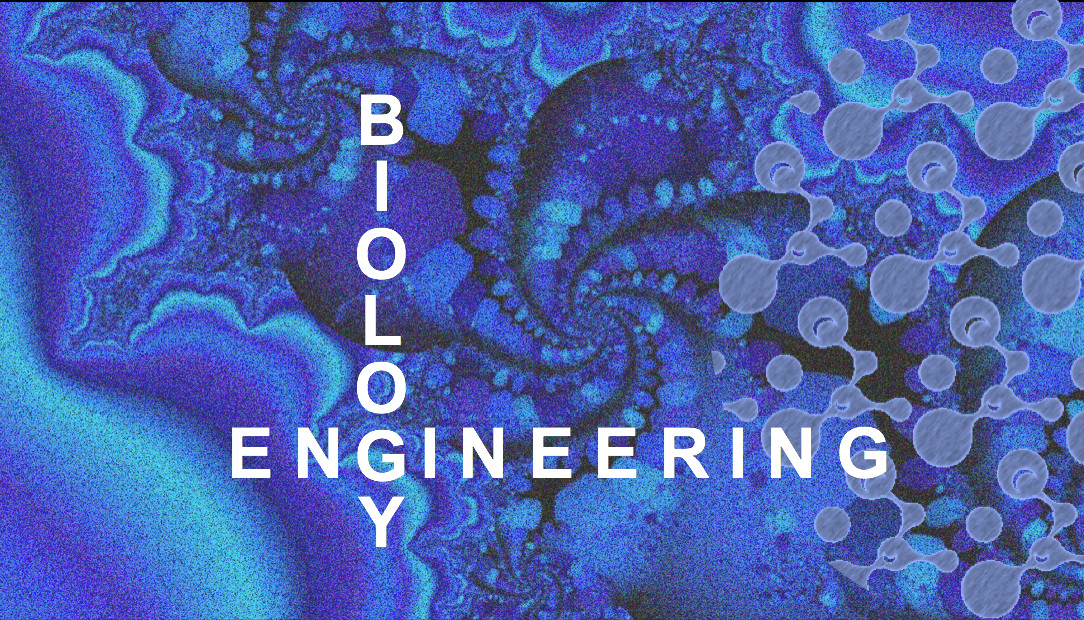
Biology and engineering – my favorite topic!
Thank you to Vijay Pande and Derek Lowe for recent blog posts discussing the influence of engineering in biology research and drug discovery.
I believe engineering principles can help us understand why drugs fail and what we can do about it, particularly if we focus on insights from complex systems engineering.
What are the relevant features of complex engineered systems?
Modularity. Modular designs create great diversity from few components (e.g. the genetic code, A T G C, cells and tissues, etc.). Modular design is highly efficient as the same component may be used for different functions. Modularity is also useful as it can function to limit damage by compartmentalizing areas of injury.
Networked architecture. Networked components can speed communication through the system, ensuring a rapid response to changes in the environment. Networked architectures provide the framework for implementing control systems that function to tune and regulate responses of the whole system. Networks often feature redundant components and produce emergent behaviors.
Control systems. Control systems dominate complex engineered systems, functioning to ensure consistent performance in uncertain environments. The more demanding the performance requirements, the greater the complexity of regulatory controls. Control system theory (design of feed-forward and feedback loops, etc.) is a major element of complex systems engineering and many comparisons have been made with regulatory motifs in biology.
Hierarchical design. Hierarchical design contributes to the diversity of system functions beyond modularity. Multiple levels of hierarchy create a combinatorial explosion in the number of structures or functions that are possible. Each level in the hierarchy (e.g. molecular, cellular, tissue, etc.) has its own network architecture with associated control systems. The structure and function of networks at higher levels of the hierarchy can’t be predicted from information at lower levels alone. Information exchange between levels is mediated by specialized components positioned at the interface.
What are the consequences of these design elements for drug discovery?
Targets can be involved in multiple biological processes. The function of a target in the disease setting may be understood but not its role in other tissues. This can lead to unexpected effects including toxicities. Many paths can lead to the same clinical outcome. This is helpful for novel target discovery, but less so for discovery of toxicity mechanisms. The hierarchical networked architecture of human biology, much of which we do not understand, makes it very difficult to predict clinical outcomes.
How can we apply these engineering principles to improve current practices in drug discovery?
Reinforce the connection to the clinic. Focus our efforts on building our knowledge of disease biology and anchoring this knowledge to the highest level of the system hierarchy – clinical outcomes (the ultimate KPI). We need stronger clinical hypotheses that provide a tighter connection between the molecular target and clinical outcome, through cellular, tissue and organ system hierarchies. There are control systems at each of these levels pushing back against drug pressure on the molecular target. Poor therapeutic hypotheses have been the downfall of many drug discovery programs – reliance on molecular pathway justifications that are vague in how they connect to disease outcomes. Studying drug failures can also help build knowledge of disease biology (see the recent commentary by Alteri & Guizzaro).
Avoid animal testing. Think about it. Human biology is different from animals in every aspect – gene sequences, protein structures, physiology, size, lifespan and environment. In networked systems small differences become amplified. Regulatory and control systems preferentially diverge. Animal data has reproducibility problems that are often hidden due to lack of transparency (highlighted by recent studies such as here and here). The more we know about disease and drug mechanisms (see also here), the more clear it becomes why animal data simply should not be relied upon for program decisions.
Advance in vitro disease models. If we are to improve productivity in drug discovery, we need better methods to build our knowledge of drug and disease mechanisms. In vitro systems that model complex tissues architectures and organ functions are advancing (see here and here). These can be exploited to help uncover control and regulatory mechanisms. Because regulatory mechanisms are often silent in the absence of stimulation, perturbations that activate these mechanisms (e.g. adaptive responses) can be used to bring them out. Many unexpected drug effects result from activation of control systems that were not represented in preclinical studies or in healthy volunteers evaluated in Phase I trials.
Biology is complicated, but not impossible, nor unpredictable. Thanks to advances in engineering and other disciplines, we have many new tools to apply to the problem. And if we pay more attention to building our understanding of human biology as a complex system we will improve success rates in drug discovery. This thinking is not new, but it is a good time for a reminder as we reconsider current animal and molecular focused approaches.