Designing Physiologically Relevant Assays
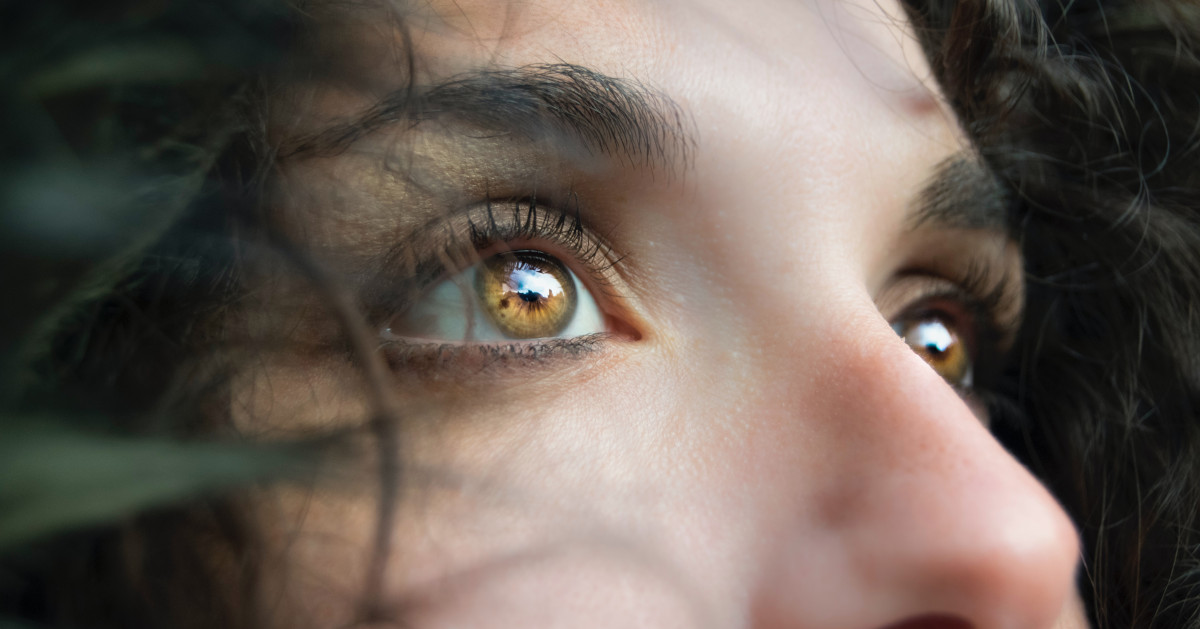
How do we design phenotypic assays that are physiologically relevant? And what do we mean by “relevant”? For those of us working in drug discovery or chemical safety, what we really want are assays that are predictive of effects in people. Easy to say but hard to do (and even harder to prove)!
Desirable features of physiologically relevant assays have been discussed over the years (see for example Vincent et al., 2015 and Horvath et al., 2016 as well as earlier work Butcher et al., 2004). My own perspective is influenced by a focus on higher throughput assays, which tend to be simpler formats.
Throughput of assays (how many samples can be tested) is important for the simple reason that to prove relevance, we need a lot of data. Only by testing many drugs can we show predictivity. While assay throughput will always be a limitation for demonstrating relevance, assays also need to have good performance metrics for reliable results. Assays that can be run at higher throughputs include two-dimensional formats (2D) and certain 3D formats (organoids), but not yet tissue chips or microphysiological systems.
Assay design features that can be optimized to improve relevance include cell type, co-cultures, assay conditions and endpoints.
Cell Type
We’ve discussed options for human cell types previously (see here). Primary cells offer the highest level of physiological relevance. Compared to cell lines and even stem cell derived cell types, primary cells retain more of the functions and regulatory pathways that are characteristic of cells in tissues. As cells are cultured, over time they lose their ability to respond normally. The longer cells are kept in cell culture (forever in the case of cell lines and months in the case of stem cell derived cell types), the more aberrant their responses become.
Co-cultures
The use of co-cultures rather than single cell types is an easy way to improve physiological relevance. Co-cultures capture the biology of cell-cell communication, an important aspect of tissue environments. Cells interact with one another through receptors as well as soluble factors to modify each other’s behavior. The use of co-cultures can also extend the utility of cell types with limited numbers.
Assay Conditions (Simulation)
Components can be added to cell cultures to create more physiologically relevant conditions. These components (or stimuli) include soluble factors, hormones, cytokines or agonists of specific receptors. We have found that combinations of stimuli are key to designing assays that are more reflective of human tissue and disease biology. Optimized combinations identified through iterative testing produce assays with unusual characteristics – displaying “robust” behaviors – reduced variability and higher reproducibility. Robustness is a feature of complex systems with highly networked architectures. You can read more about biological complexity here or in this this previous post.
Endpoints
The most desirable assay endpoints are those that are closest to clinical outcomes. Cell surface biomarkers and secreted metabolites or factors can be readily tracked in clinical studies. Biomarkers that are known clinical risk factors have the advantage that their disease association has already been established. Endpoints such as cell death or intracellular pathway intermediates are not as easily translatable to disease outcomes.
The assay features described above and the number of possible combinations of cell types, formats, assay conditions, and endpoints may seem overwhelming. However, we should not forget that iterative testing of different combinations, along with large scale testing of drug effects can be a powerful way to build our understanding of disease and tissue biology. This is time and effort well spent.
Photo credit: Marina Vitale on Unsplash