Catalyzing change at the NIH with Novel Alternative Methods
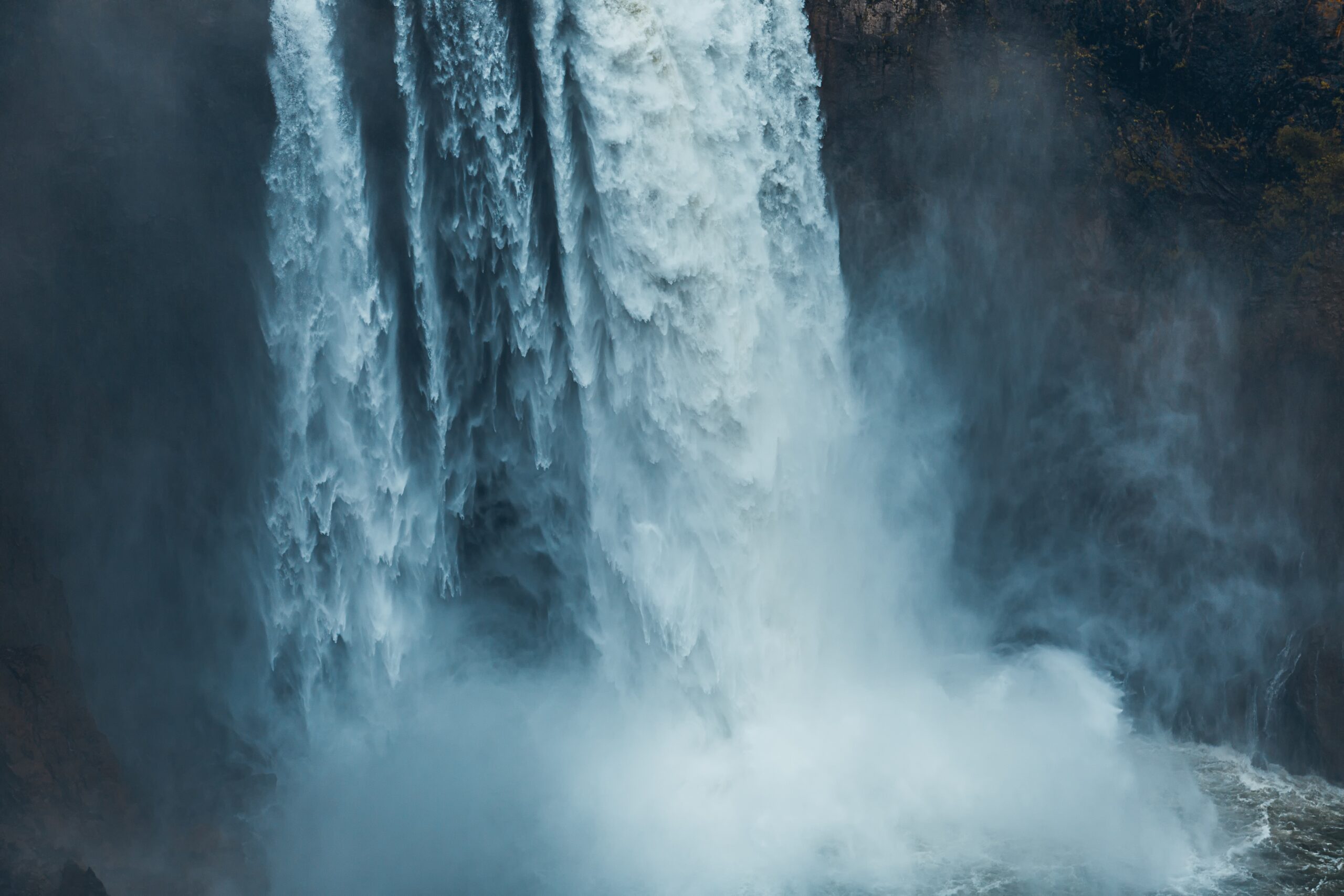
The NIH’s workshop on “Catalyzing the Development and Use of Novel Alternative Methods” brought together a diverse group of researchers and leaders to address one of the largest challenges in biomedical research today – how do we move on from our dependence on animal-based research.
Moving on from animal studies to embrace more human-relevant approaches will not be easy. In the past, biomedical research has benefited from animal models to discover and understand basic biological mechanisms. For myself, animal experimentation was critical for the discovery and understanding of lymphocyte homing receptors (Streeter, 1988; Nakache, 1989; Berg, 1991), and development of novel biotherapeutics (He, 1998; Haring, 1996).
But the world has changed. We have advanced our baseline understanding of human disease biology. We have new data sources and new tools – new methods for omics measurements, single cell analysis and cell culture. And because of this, our high-priority research questions have changed. These questions are no longer ones that can be effectively addressed by traditional animal model-based research.
Through efforts such as the NIH’s All Of Us program, UK Biobank, and many others, we now have large cohort databases containing patient records, genetic and other omics data, exposure info and more. These data have created a flood of new information that is rapidly accelerating our understanding of disease biology and human health, leading us closer to the reality of precision medicine.
Capitalizing on clinical cohort data will be one of the highest priorities in biomedical research. To be successful, the NIH has recognized that “Novel Alternative Methods” or NAMs are key. Human relevant NAMs, including in silico, in chemico (e.g., biochemical) and in vitro (e.g., cell based) systems, can directly map these new information sources. Human-based NAMs allow for rapid testing and confirmation of hypotheses generated from large patient-level data sets. And these NAMs themselves can be used to generate high value data sets for incorporating into predictive models and AI/ML approaches.
The future in biomedical research will be (even more) data centric. So, our priorities should include quality management and infrastructure systems that support biomedical data-driven research. This means production level data management systems and stronger data governance processes. We also need better alignment on standards for experimental documentation, for assay performance characterization and for metadata management. And while high quality, well-annotated data are important, we also need to make sure data and tools are FAIR: findable, accessible, interoperable (or interpretable) and reusable. FAIR principles are foundational to a productive and equitable research community.
We look forward to working with interested colleagues on projects to advance the development and use of NAMs in biomedical research.
Photo by Stephen Walker on Unsplash.