Can Network Biology Fuel Innovation in Drug Discovery?
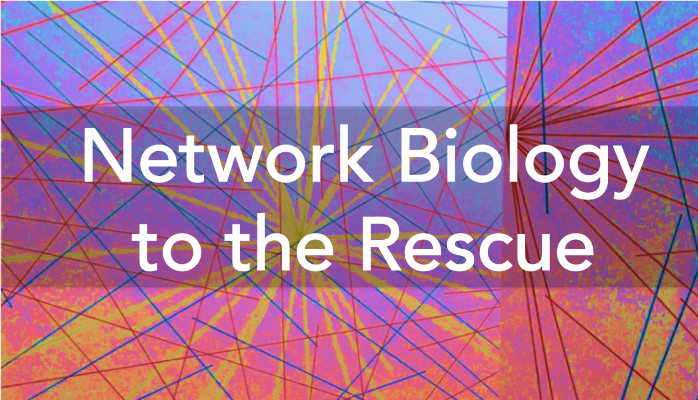
If phenotypic screening is driving innovation in drug discovery, network biology is the fuel.
In phenotypic screening, compounds are tested for their functional effects on cellular responses in a target agnostic manner. For drug discovery programs, not knowing the target is a risk. The target may not be novel, or it may be associated with some toxic side effect. Thus, advances in techniques for mechanism of action deconvolution and target identification are of great interest to phenotypic drug discovery researchers.
A number of methods, both wet lab and computational, for compound mechanism of action were presented at the recent Keystone Symposium on Phenotypic Drug Discovery (PDD). In the session on target identification, chaired by John Moffat(Genentech), Monica Schoene (Broad Institute) described direct biochemical approaches using affinity enrichment combined with quantitative mass spectrometry. Among other examples, this method was the basis of the successful identification of an E3 ubiquitin ligase (containing CRBN and DDB1) as the target of the multiple myeloma drug, lenalidomide.
The challenges of biochemical methods and importance of integrated approaches were underscored by Suzanne Swalley (Novartis), who also spoke in this session. She presented the tour de force story of the identification of the U1 snRNP complex as the target of LMI070, a novel small molecule drug in clinical trials for the treatment of patients with spinal muscular atrophy. This took an extraordinary effort, as indicated by the 40 authors on the resulting paper.
In silico approaches for identifying compound targets were presented by Andreas Bender (University of Cambridge) and Vladimir Chupakhin (Janssen). Computational methods use known relationships between chemical structure, targets, gene expression and/or phenotypic endpoints to make predictions. For this, a variety of data sources and techniques can be employed, including decision trees and deep learning methods such as artificial neural networks and matrix factorization. As with the biochemical methods, these too are most effective when applied as part of an integrated strategy.
Andrea Califano (Columbia) demonstrated how network biology effectively combines results from both biochemical methods and computational predictions to generate molecular interaction networks. These networks, in conjunction with drug perturbed gene expression profiling data sets, have been applied to infer mechanism of action targets, for example, using the DeMAND algorithm that tests for dysregulated network interactions. Califano also described how these inference methods applied to identify upstream protein targets controlling key cancer targets have revealed a regulatory feedback pathway for the breast cancer target Her2 (ERBB2) involving IL-6R/Jak.
It is this last example that illustrates the value of network biology. Not only can network approaches contribute to drug target identification, but they can also reveal important pathway mechanisms that are critical for developing efficacious drugs. Furthermore, when we apply network biology and related systems biology methods to the cellular assays used in phenotypic screening programs, details of the underlying complex architecture of the biological model systems are revealed. This knowledge improves our overall understanding of disease biology, and by reducing the risk of the unknown, increases our confidence in the resulting drug candidates.
Let the adventure continue!
For other viewpoints from the conference, see here, here, here, and here. And be sure to check in with the SLAS Phenotypic Drug Discovery Special Interest Group for the latest discussions.