Assay Endpoints – Measure What Matters
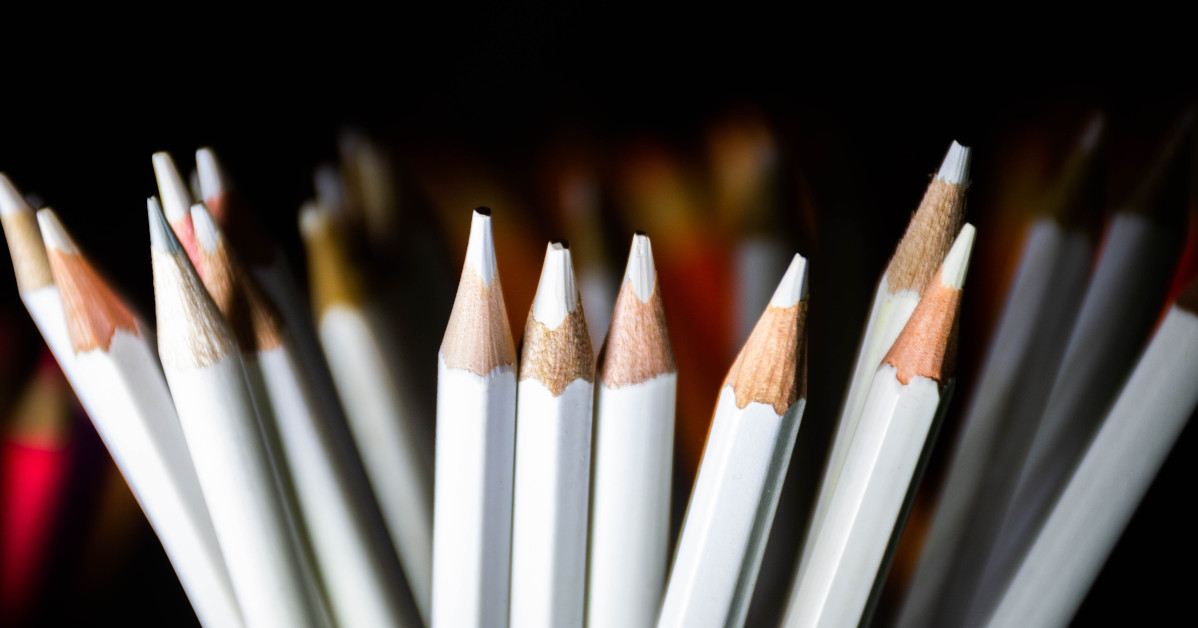
It’s not just about measuring what matters – it’s about making what you measure matter.
There are many options for endpoints to measure in phenotypic assays. Endpoints to consider include cell cytotoxicity, proliferation, intracellular signaling intermediates, cell surface or secreted proteins, metabolites and gene expression. More complex outputs such as imaging or electrical current-based (impedance) measurements could also be selected. The choice of what to measure will depend on access to detection technologies, cost considerations as well as the research team’s analytical capabilities. Assays with complex outputs will require specialized expertise in bioinformatics or image analysis.
To choose the best option, however, researchers should also consider the limitations and consequences of their choice. For results to be accurately and effectively interpreted, different follow-up assays will be required.
Cell cytotoxicity is a common endpoint for oncology and many toxicity assays. These can be inexpensive endpoints to measure using, for example, metabolic dyes to capture cell metabolism (e.g., Alamar Blue or Resazurin) or protein binding dyes for adherent cell types (e.g., Sulforhodamine B). For oncology programs, counterscreens against normal cell types will be needed to ensure selectivity for tumor cells. Many different mechanisms lead to cell death, so follow up assays to determine mechanism of action may be needed. Note that use of cytotoxicity assays for safety/toxicity applications can be challenging. Cytotoxicity of cell types in vitro are not very predictive of in vivo effects. This is because many important toxicity mechanisms do not cause overt cell cytotoxicity in vitro (e.g., arrhythmia, cardiovascular, nausea, etc.).
Pathway-based screens measure intracellular signaling intermediates as endpoints. These screens may use reporter genes or molecular sensors to measure levels of phosphorylated forms of signaling proteins or levels of metabolites to indicate pathway or organelle states (e.g., mitochondria membrane potential, etc.). The main limitation of these endpoints is their uncertain translation. Effects at the pathway level often do not translate to effects at the tissue or organ level of biology (e.g., due to feedback mechanisms). This is because pathway activities are highly influenced by cell type and cell state (for example, inflammatory, nutrient deprived conditions, etc.). Follow up assays will need to include other cell types and states as well as orthogonal studies in more disease relevant assays.
Assays that are more disease relevant often measure the levels of cell surface proteins or secreted molecules using antibody-based technologies, for example by ELISA or flow cytometry. While these endpoints can be expensive to measure, they are more proximal to clinical outcomes than intracellular signaling intermediates. Indeed, many of these molecules are established clinical biomarkers. This facilitates translation of assay results to in vivo efficacy or toxicity outcomes.
Endpoints that are comprised of complex multi-dimensional outputs include high content imaging (including Cell Painting), gene expression, and electrical current or impedance-based endpoints. These complex outputs have been useful for disease screens, where there is a clear distinction between normal and disease patterns. The limitation of these outputs for other applications is in the interpretation of patterns for mechanism of action determination. Interpretation of different patterns typically requires a reference dataset and assays with sufficient reproducibility. These methods also require significant data management and analytics expertise. Data sets can get very large, and methods to interpret and classify patterns require expertise in machine learning and statistics.
Gene expression-based endpoints can support clinical translation, as patterns can be matched to those measured in clinical studies. Although gene expression is more distal to tissue and organ level biology than protein endpoints, there are advantages. Measurement technologies have advanced to minimize sample costs and increase scale (e.g., RNA-Seq). Indeed advances in single cell transcriptomics (scRNA) and associated analysis methods (for example PopAlign) may improve the interpretability of gene expression profiles.
When choosing which endpoint type to measure, it is important to keep in mind what follow up studies will be needed to accurately interpret results. In drug discovery, no single assay stands alone. Data from multiple assays must be integrated to support effective decisions. It’s not just about measuring what matters – it’s about making what you measure matter.
Photo by Lucas George Wendt on Unsplash