AI in Drug Discovery – Why We Are Not There Yet
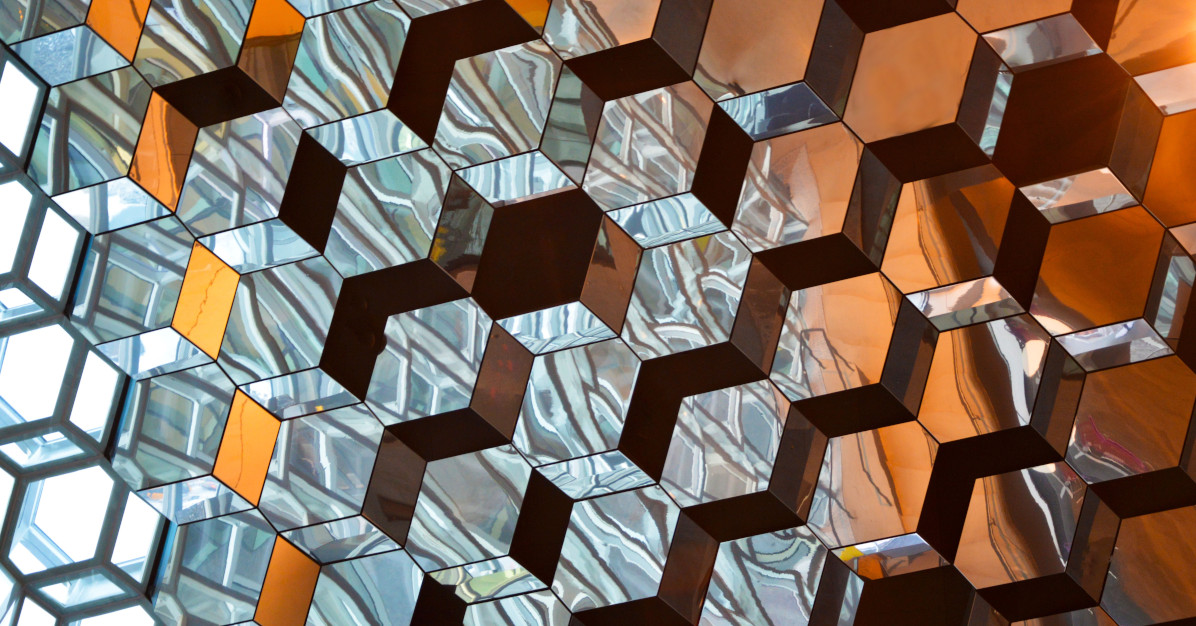
Check out the thoughtful and comprehensive review of AI in drug discovery by Andreas Bender and Isidro Cortés-Ciriano, Artificial intelligence in drug discovery: what is realistic, what are illusions? Part 1: Ways to make an impact, and why we are not there yet and part 2, Artificial intelligence in drug discovery: what is realistic, what are illusions? Part 2: a discussion of chemical and biological data.
TL;DR
Created by the author in Canva.
Applications for AI in chemistry have been more successful than in biology. In chemistry, the data we have (so-called “proxy measures”) are on endpoints that are close to the outcomes we care about. For example, to predict whether or not new compounds will bind to a protein target. This is not true for most biology applications. For these, the data we have are on endpoints far away from the outcomes we care about. For predicting drug safety or efficacy, we have data on proxy measures that are only very distantly connected to these outcomes. This problem is further exacerbated by insufficient training data (i.e., from successful and failed drugs). To address the challenge of predicting drug safety and efficacy, alternative approaches including mechanism based tools such as AOP and DPOP frameworks may help (see here and here as well as the post here).
From the article:
“When it comes to data supporting the early stages of drug discovery, we need to realize that the data we currently have are unlikely to give us the answer needed to judge the efficacy and safety of a compound . . . Only understanding biological end points of relevance will allow us to generate the data needed for quality-based decision making, which is required to make drug discovery overall more successful.”
“To truly advance the field, and to go from applications in ligand discovery to those in drug discovery (beyond developing chemical probes, which of course can be useful for target validation by themselves for example), we need to understand which data to generate for which purpose, and this involves understanding biology better in the first place. Only when we are then able to measure and capture relevant biological endpoints in vivo we will be able to advance the field significantly further, and to apply the computational algorithms currently available to us fruitfully in the drug discovery area, with respect to compound efficacy and safety in the clinic.”
Photo by Christelle BOURGEOIS on Unsplash.