Phenotypic Screening – Advancing the Science
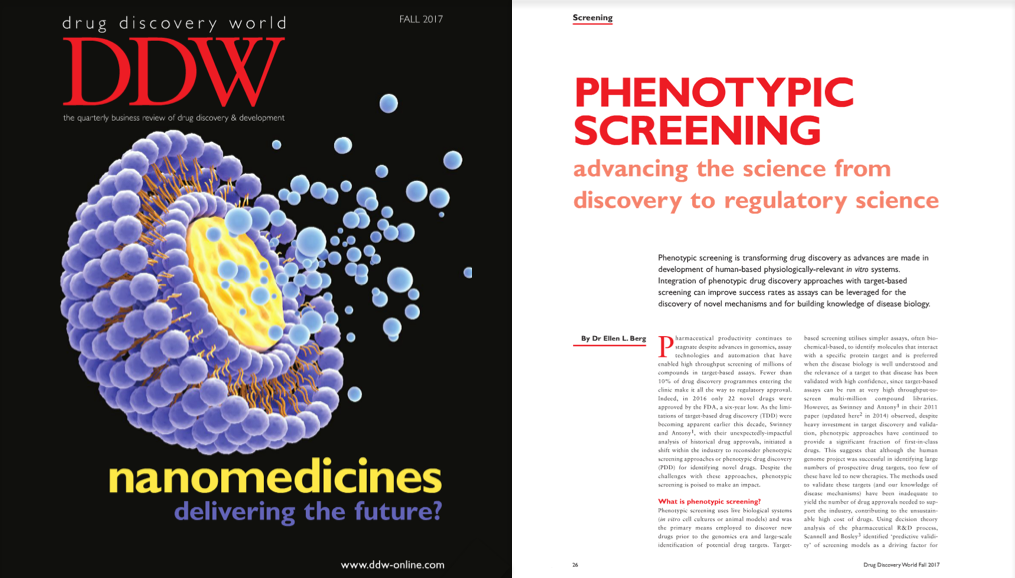
This new article in DDW adds to some very good recent perspectives on phenotypic drug discovery (PDD) from the pharma industry in NRDD and ADDT (see additional articles and further commentary here, here and here) that lay out the current situation, challenges, and lessons learned in applying phenotypic screening in drug discovery. This new piece is focused more on the future, on what is needed to fulfill the promise of PDD and how can we address the central issue in the industry – improving pharmaceutical research productivity.
The perspective by John Moffat (Genentech), Fabien Vincent (Pfizer), Jonathan Lee (Eli Lilly), Jörg Eder (Novartis) and Marco Prunotto (Roche), “Opportunities and challenges in phenotypic drug discovery: an industry perspective“, is informed by the collective experiences of a diverse group of pharmaceutical researchers and walks through the core concepts of phenotypic drug discovery (PDD). It presents many of the cautions, but also includes practical recommendations and future directions. Particularly useful is their concept of “chain of translatability” which emphasizes the need for phenotypic assays (as is true for target-based assays) to be “mechanistically informed” and validated for disease relevance. This paper also points out molecular phenotyping and advanced cellular models such as organs-on-a-chip as providing potential solutions for the future.
Advanced cellular models, however, are experiencing their own “hype cycle”. Despite the excitement of recent headlines (see here and here), we are not there yet. The development of these complex systems, including 3D models, bioprinted tissues, and microphysiological systems or tissue chips, is in many ways still in the early phases. Their development and validation is subject to the challenge of combinatorial complexity: as the numbers of components in a system increase, the corresponding numbers of combinations escalate exponentially. The work required to test all of the potential variables is substantial. Indeed, my own team’s effort to develop a “simple” model of wound healing consisting of a single cell type, human primary fibroblasts (the BioMAP HDF3CGF system), took more than 3 years with evaluation of 1000s of permutations. The final conditions selected include a specific cocktail of six pathway activators, all six of which were required to achieve a stable cell signaling network state that accurately modeled the human tissue pathophysiology of wound healing, was sufficiently reproducible across experiments and donors (robust), and responded as expected to known perturbations. If we then consider the desire to combine cell types, modify spatial and mechanical features, and connect systems to one another, the number of permutations (as well as costs and timelines) quickly becomes overwhelming. But don’t misunderstand my caution. Despite these challenges, supporting the development of these systems is incredibly important and highly valuable. Not only do these systems have the potential for use in evaluating new drug leads for efficacy or in chemical safety testing, but the process itself of developing these systems will teach us much about basic mechanisms of human biology along the way. In this, the journey is as valuable as reaching the destination.
The recent perspective by Dorothea Haasen and colleagues from Novartis (NIBR), “How Phenotypic Screening Influenced Drug Discovery: Lessons from Five Years of Practice”, gives a historical accounting of one company’s experiences with implementing phenotypic screening assays as a strategy for new drug discovery. They, too, point out the need for an in-depth understanding of the underlying biology and emphasize the development of a high-confidence phenotype-compound pair as a key success factor. The Novartis team seems to be more comfortable than Moffat et al. with leaving target deconvolution to later stages of a project (Novartis is known among pharma companies for its strength in biology). In their article, Haasen et al. advocate for extended assay prototyping and execution of small-to-medium sized pilot screens to learn as much as possible about the disease biology at an early stage. By following this approach, they can gain a more informed understanding of the likely novelty of their compounds and potential risks, even in the absence of a defined target.
Both the Moffat and the Haasen papers highlight the requirement for larger up-front investments by phenotypic programs compared to target-based programs. This suggests that in order for the pharma industry to justify taking on phenotypic approaches for new drug discovery, these approaches must deliver a higher rate of success than current target-based drug discovery (<10% of programs that enter clinical testing lead to an approved drug). Otherwise, the return on investment (ROI) will not be justified. While everyone agrees that improving a program’s likelihood of success requires a validated disease hypothesis, the path for achieving this is not so clear.
This is the subject taken on in the DDW perspective. How can we leverage investments in phenotypic approaches to improve productivity? This article advocates for a multi-pronged approach, mapping out the known disease pathophysiology at the cellular/tissue level (using an outcome pathway framework) and simultaneously exploring multiple phenotypic and target-based screens. This recommendation follows in part the lead of the Novartis group, building disease knowledge through small, information-gathering pilot studies. The outcome pathway framework is reminiscent of the “chain of translatability” concept from the Moffat paper, as well as the Adverse Outcome Pathway concept from chemical safety testing (sans animal models, see here for why this is my position). While this approach takes time and resources, success rates will be higher (from improved knowledge of disease biology and pursuing multiple hypotheses simultaneously). The key value driver from taking this approach derives from leveraging the resulting chemical biology datasets to improve in silico cheminformatics as well as to tackle the problem of drug toxicity mechanisms (see more here and here).
As is true for any important endeavor, the path forward will not be easy. It requires even higher levels of cross-disciplinary collaboration than we have yet seen, a focus on biology first, as well as strong leadership and commitment. Are we up for it?